
In the world of data science, time series analysis plays a crucial role in uncovering patterns and extracting valuable insights from temporal data. With the advancement of technology and the ever-increasing volume of data, businesses and organizations are seeking innovative ways to harness the power of time series analysis to make accurate predictions and drive informed decision-making.
Understanding Time Series Analysis
Before diving into the specifics of using data snapshots for predictive insights, let's first explore the fundamentals of time series analysis. At its core, time series analysis is a statistical method used to analyze and forecast data that changes over time. By identifying patterns, trends, and anomalies within a dataset, analysts can gain a deeper understanding of the underlying factors influencing a particular phenomenon.
Time series data is characterized by its sequential nature, where each data point is recorded at regular intervals. This sequential structure allows analysts to uncover dependencies and relationships between observations, providing valuable insights into how variables evolve over time. Understanding these dynamics is crucial for making informed decisions and developing accurate predictive models.
The Basics of Time Series Analysis
Time series analysis involves a series of steps to extract meaningful information from temporal data. It begins with data collection, followed by data cleaning and preparation. Once the data is in a suitable format, analysts can apply various statistical techniques, such as autoregressive integrated moving average (ARIMA) models and exponential smoothing methods, to uncover hidden patterns and make predictions.
Exploring the seasonality, trends, and cyclical patterns within time series data is essential for capturing the underlying dynamics of a system. Seasonal variations, for example, can provide insights into recurring patterns that influence the data at specific time intervals. By accounting for these patterns, analysts can improve the accuracy of their forecasts and make more informed decisions based on historical trends.
Importance of Time Series Analysis in Data Science
Time series analysis is a critical tool in data science, as it enables decision-makers to understand the dynamics of a system and predict future outcomes. From financial forecasting to demand planning and forecasting natural phenomena, time series analysis provides valuable insights that can drive strategic decision-making and improve operational efficiency.
Furthermore, time series analysis plays a crucial role in anomaly detection, where deviations from expected patterns can signal potential issues or opportunities. By monitoring and analyzing time series data, organizations can proactively identify outliers and unusual behavior, allowing them to take timely actions to mitigate risks or capitalize on emerging trends. This proactive approach enhances the overall resilience and adaptability of businesses in an ever-changing environment.
The Role of Data Snapshots in Time Series Analysis
Now, let's delve into the integral role data snapshots play in the realm of time series analysis. A data snapshot is a collection of data captured at a specific moment in time. It serves as a representation of the state of a system, allowing analysts to examine historical data and perform analyses with a higher level of precision.
Defining Data Snapshots
Data snapshots consist of a wide range of data points, such as stock prices, temperature measurements, or website traffic statistics, collected at regular intervals. These snapshots serve as building blocks for time series analysis, providing a comprehensive view of the variables under scrutiny.
How Data Snapshots Contribute to Time Series Analysis
By utilizing data snapshots, analysts can incorporate the element of time into their analyses, capturing the temporal variations that occur within a dataset. This temporal dimension enables the identification of patterns, trends, and seasonality in the data – essential components for accurate forecasting. Furthermore, data snapshots allow analysts to evaluate the impact of external factors and events on the observed phenomenon, thereby enhancing the predictive power of the analysis.
Steps in Conducting Time Series Analysis
Now that we have established the significance of time series analysis and the role of data snapshots, let's outline the key steps involved in conducting a successful time series analysis:

Data Collection for Time Series Analysis
The initial step in any time series analysis is to gather relevant data. This involves identifying the variables of interest, determining the appropriate frequency and duration of data collection, and ensuring data integrity through robust data collection methods.
Data Cleaning and Preparation
Once the data is collected, the next step is to clean and prepare it for analysis. This involves handling missing values, addressing outliers, and transforming the data to ensure it meets the assumptions of the selected analytical techniques. Data cleaning is crucial in ensuring the accuracy and reliability of the subsequent analysis.
Implementing the Analysis
With the data prepared, analysts can then apply various time series analysis techniques to extract insights. This may involve applying statistical models, identifying patterns through visualizations, or harnessing machine learning algorithms to make predictions. The choice of technique depends on the nature of the data and the specific research or business objective.
Predictive Insights from Time Series Analysis
At the heart of time series analysis lies the ability to generate predictive insights based on historical data. These insights allow decision-makers to anticipate future trends, mitigate risks, and optimize resource allocation.
Interpreting Results from Time Series Analysis
Interpreting the results of a time series analysis is a complex task, often requiring a blend of statistical expertise and domain knowledge. Analysts must carefully scrutinize the identified patterns and trends, making sure they align with the objectives of the analysis. By critically assessing these results, decision-makers can make informed choices and develop effective strategies.
Making Predictions Based on Time Series Analysis
One of the primary objectives of time series analysis is to make accurate predictions for future outcomes. By leveraging the historical patterns identified, analysts can develop forecasting models that estimate future values with a certain degree of confidence. This helps organizations proactively plan and allocate resources, optimizing their operations for maximum efficiency.
Challenges and Solutions in Time Series Analysis
While time series analysis offers a world of possibilities, there are inherent challenges that analysts must navigate to ensure the reliability and accuracy of their predictions.

Common Pitfalls in Time Series Analysis
One common pitfall in time series analysis is the presence of outliers or anomalies that can skew the results. These anomalies could be due to erroneous data, unexpected events, or missing values. It is crucial for analysts to identify and handle these outliers appropriately to avoid misleading conclusions.
Overcoming Challenges in Time Series Analysis
To overcome the challenges in time series analysis, analysts employ various techniques such as outlier detection algorithms, imputation methods for missing data, or data transformation approaches. Additionally, employing ensemble models, combining multiple methodologies, can help improve the accuracy and robustness of predictions.
Time series analysis combined with the power of data snapshots offers a potent framework for unlocking future insights. By understanding the fundamental concepts, leveraging data snapshots effectively, and employing sound analysis techniques, organizations can harness the power of time series analysis to gain predictive insights and drive informed decision-making. So, why rely on guesswork when you can rely on data?
Unlock the Future of Your Business with Scoop Analytics
Ready to transform your time series analysis and gain predictive insights with ease? Scoop Analytics is here to revolutionize the way you approach data-driven decision-making. With our user-friendly snapshotting feature, you can effortlessly capture the essence of your data over time, ensuring you never miss a beat in your business's performance. Say goodbye to the complexities of coding and manual data tracking, and hello to a world where strategic insights are just a snapshot away. Don't let the opportunity to enhance your decision-making slip through your fingers. Try Scoop free today and start making the most of your data with confidence.
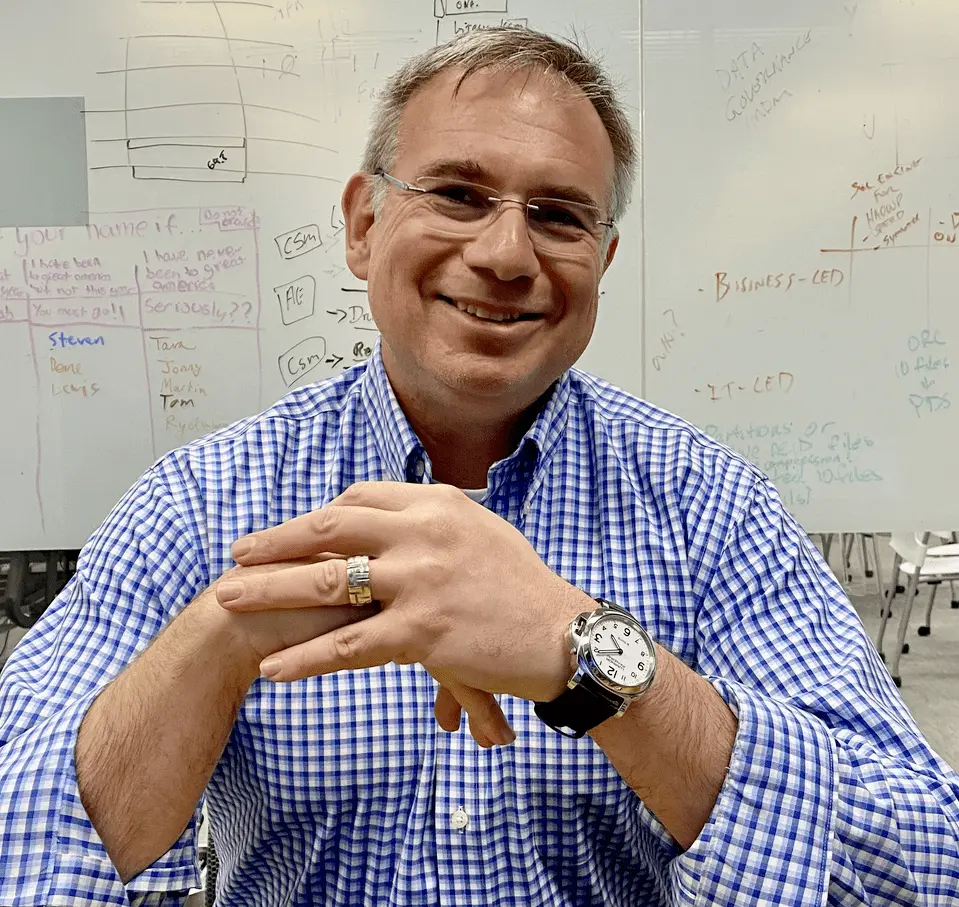